IBM SPSS AMOS 結構方程模型分析軟體
- IBM SPSS AMOS 結構方程模型分析軟體
-
類別統計分析軟體
-
介紹IBM SPSS AMOS 是一款專業的結構方程模型(SEM)分析軟體,可用於數據分析、統計建模、因果關係分析等。該軟體基於最新的結構方程建模技術,可幫助用戶構建複雜的模型並進行分析。您可以繪製結構方程模型圖、設置模型參數、模型適配度檢測、多樣本比較等操作。該軟體支持多種分析方法,如路徑分析、因果關係分析、驗證性因子分析、多層次建模等,幫助用戶從數據中發現深層次的因果關係和影響因素。此外,IBM SPSS AMOS 還提供了豐富的圖表和報告生成工具,使得用戶可以直接地呈現和分享研究成果。如果您是一名統計學家、社會科學研究人員、市場調研人員或學術人員,IBM SPSS AMOS 將是您不可或缺的工具。
IBM SPSS Amos 29
IBM SPSS Amos was designed as a tool for teaching structural equation modeling in a way that emphasizes the simplicity that underlies this powerful approach to data analysis. Every effort was made to see that it is easy to use. Amos integrates an easy-to-use graphical interface with an advanced computing engine for SEM. The publication-quality path diagrams of Amos provide a clear representation of models for students and fellow researchers. The numeric methods implemented in Amos are among the most effective and reliable available.
Graphical and non-graphical model specification
IBM SPSS Amos accepts a path diagram as a model specification and displays parameter estimates on a path diagram. Path diagrams used for model specification and those that display parameter estimates are of presentation quality. They can be printed directly or imported into other applications such as word processors, desktop publishing programs, and general-purpose graphics programs.
Full information estimation with missing data
When some data values are missing, Amos offers a choice between maximum likelihood estimation or Bayesian estimation instead of ad hoc methods like listwise or pairwise deletion.
Bootstrap
The program makes bootstrap standard errors and confidence intervals available for all parameter estimates, effect estimates, sample means, variances, covariances, and correlations. It also implements percentile intervals and bias-corrected percentile intervals (Stine, 1989), as well as Bollen and Stine’s (1992) bootstrap approach to model testing.
Model comparisons
Multiple models can be fitted in a single analysis. Amos examines every pair of models in which one model can be obtained by placing restrictions on the parameters of the other. The program reports several statistics appropriate for comparing such models.
Group comparisons
Amos can analyze data from several populations at once.
Bayesian estimation
Amos uses an MCMC (Markov chain Monte Carlo) algorithm to perform Bayesian estimation. Bayesian estimation can be used to to avoid negative variance estimates and other types of improper solutions. It can be used to estimate any arbitrary function of the model parameters.
Estimation of means and intercepts
Amos makes it easy to estimate means for exogenous variables and intercepts in regression equations.
Specification search (Exploratory SEM)
Amos's specification search provides a method for systematically specifying, fitting and evaluating a large number of candidate models. In a specification search, some single- and double- headed arrows in a path diagram are designated as optional. Amos fits the model both with and without each optional arrow, using every possible subset of the optional arrows. Tools are provided for choosing among the models on the basis of fit, parsimony, and interpretability.
Data imputation
If your dataset contains missing values, you can use regression imputation to create a new, completed dataset in which the missing values have been filled in with estimated numeric values.
In addition, if your dataset contains missing, censored, or ordered-categorical values, you can use either Bayesian imputation or stochastic regression imputation to create one or more completed datasets in which the missing, censored or ordered-categorical values have been filled in with estimated numeric values.
Analysis of censored data
Amos uses Bayesian estimation to fit models to datasets that contain censored values. It can estimate posterior predictive distributions for censored values, and perform imputation for censored values.
Analysis of ordered-categorical data
Amos uses Bayesian estimation to fit models to datasets that contain ordered-categorical variables. (For example, variables that are scored pass/fail or low/medium/high rather than numerically.) It can estimate the posterior predictive distribution of the numeric variable that underlies a categorical response, and can impute a numeric value for a categorical response.
Mixture modeling
Mixture modeling is appropriate when you have a model that is incorrect for an entire population, but where the population can be divided into subgroups in such a way that the model is correct in each subgroup. Amos allows (but does not require) you to assign some cases to groups before the analysis starts. Amos attempts to learn from any cases that are already classified and then to classify those cases that are not already classified.
User-defined estimands
Amos can estimate any function of the model parameters, complete with bootstrap standard errors, confidence intervals and significance tests.
Growth curve analysis
Amos can construct linear growth curve models.
Tests of assumptions
Amos provides a test of univariate normality for each observed variable as well as a test of multivariate normality. It attempts to detect outliers.
Annotated text output
Text output is annotated by popups that provide brief explanations of selected portions of the output.
Extensive online help.
Extensive context-sensitive help is supplied with the program in Microsoft's CHM format (the usual help format for Windows programs). The same help content is provided on this website in a more web-friendly format.
User's guide with many examples
39 examples in the user's guide show how to use Amos.
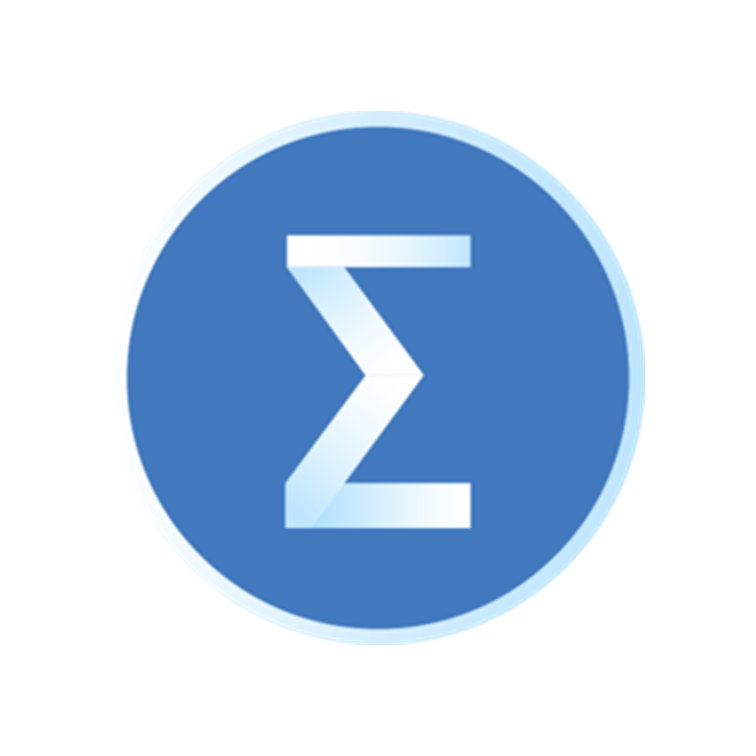
IBM SPSS Amos 29 系統需求
Windows 10, Windows 11, Windows Server 2012 R2, 2016, 2019
CPU
Intel or AMD x86 processor running at 1 gigahertz (GHz) or higher
RAM
2 gigabyte (GB) of RAM or more.
HD
1 gigabytes (GB) of available hard-disk space.
Display
XGA (1024x768) or a higher-resolution monitor
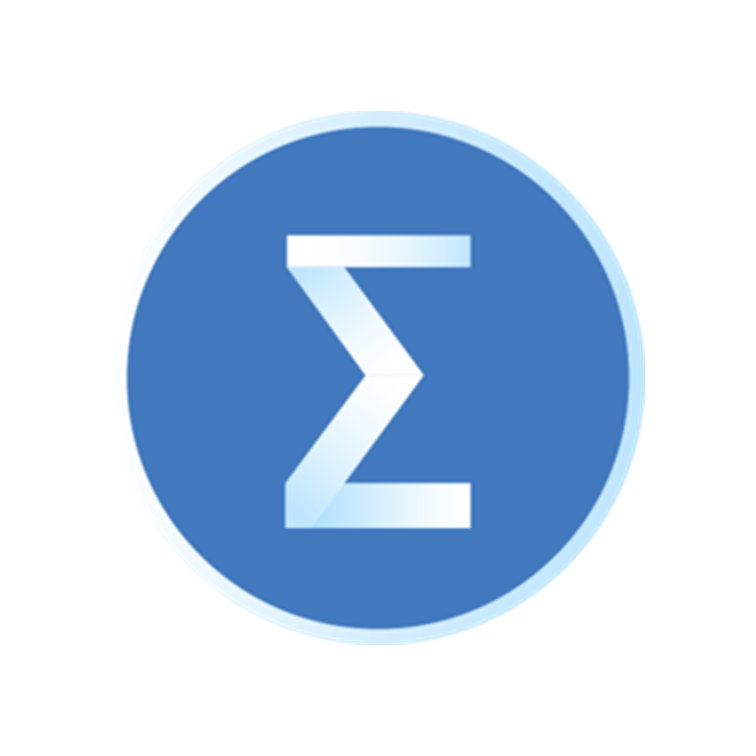
IBM SPSS Amos 29 結構方程模式軟體
IBM SPSS Amos被設計為用於教學結構方程式建模的工具,其強調了這種強大的數據分析方法所基於的簡單性。我們竭盡全力發現它易於使用。Amos將易於使用的圖形界面與用於SEM的高級計算引擎集成在一起。Amos的出版質量路徑圖為學生和研究人員提供了清晰的模型表示。在Amos中實現的數字方法是最有效,最可靠的方法之一。
圖形和非圖形模型規範
IBM SPSS Amos接受路徑圖作為模型規範,並在路徑圖上顯示參數估計。用於模型說明的路徑圖和用於顯示參數估計值的路徑圖具有表示質量。它們可以直接打印或導入到其他應用程序中,例如文字處理器,桌面發布程序和通用圖形程序。
缺少數據的完整信息估計
當缺少某些數據值時,Amos可以在最大似然估計或貝葉斯估計之間進行選擇,而不是像逐列表刪除或逐對刪除這樣的臨時方法。
引導程序
該程序使自舉標準誤差和置信區間可用於所有參數估計,效果估計,樣本均值,方差,協方差和相關性。它還實現了百分位區間和偏差校正的百分位區間(Stine,1989),以及Bollen和Stine(1992)的引導方法進行模型測試。
模型比較
可以在一個分析中擬合多個模型。Amos檢查每對模型,可以通過對另一模型的參數施加限制來獲得一個模型。該程序報告了一些適用於比較此類模型的統計信息。
組比較
Amos可以一次分析來自多個總體的數據。
貝葉斯估計
Amos使用MCMC(馬爾可夫鏈蒙特卡洛)算法執行貝葉斯估計。貝葉斯估計可用於避免負方差估計和其他類型的不正確解決方案。它可用於估計模型參數的任意函數。
均值和截距的估計
通過Amos,可以輕鬆估算回歸方程中外生變量和截距的均值。
規格搜索(探索型SEM)
Amos的規範搜索提供了一種系統地指定,擬合和評估大量候選模型的方法。在規格搜索中,路徑圖中的某些單頭和雙頭箭頭被指定為可選。Amos使用可選箭頭的每個可能子集,對帶有或不帶有每個可選箭頭的模型進行擬合。提供了用於根據擬合度,簡約性和可解釋性在模型之間進行選擇的工具。
數據插補
如果您的數據集包含缺失值,則可以使用回歸插補來創建新的完整數據集,在該數據集中,缺失值已用估計的數值填充。
此外,如果您的數據集包含缺失,檢查或有序分類值,則可以使用貝葉斯插補或隨機回歸插補來創建一個或多個完整的數據集,在其中填充了缺失,檢查或有序分類值估計數值。
審查數據分析
Amos使用貝葉斯估計將模型擬合到包含檢查值的數據集。它可以為刪失值估計後驗預測分佈,並為刪失值執行估算。
有序分類數據分析
Amos使用貝葉斯估計將模型擬合到包含有序分類變量的數據集。(例如,得分為通過/失敗或低/中/高而不是數字的變量。)它可以估計作為類別響應基礎的數字變量的後驗預測分佈,並可以為類別響應推算數值。
混合物建模
當您使用的模型對於整個總體而言都是不正確的,但可以將總體劃分為子組時,每個模型中的模型都是正確的,則可以使用混合建模。在分析開始之前,Amos允許(但不要求)將一些個案分配給組。Amos嘗試從已經分類的所有案例中學習,然後對尚未分類的那些案例進行分類。
用戶定義的估計
Amos可以估計模型參數的任何功能,並具有自舉標準誤差,置信區間和顯著性檢驗。
增長曲線分析
Amos可以構建線性增長曲線模型。
假設檢驗
Amos提供了針對每個觀察到的變量的單變量正態性檢驗以及多變量正態性檢驗。它試圖檢測離群值。
帶註釋的文本輸出
文本輸出由彈出窗口註釋,這些彈出窗口提供對輸出的選定部分的簡要說明。
廣泛的在線幫助
該程序以Microsoft的CHM格式(Windows程序的常用幫助格式)提供了廣泛的上下文相關幫助。本網站上以網絡更友好的格式提供了相同的幫助內容。
帶有許多示例的用戶指南
用戶指南中的39個示例顯示瞭如何使用Amos。
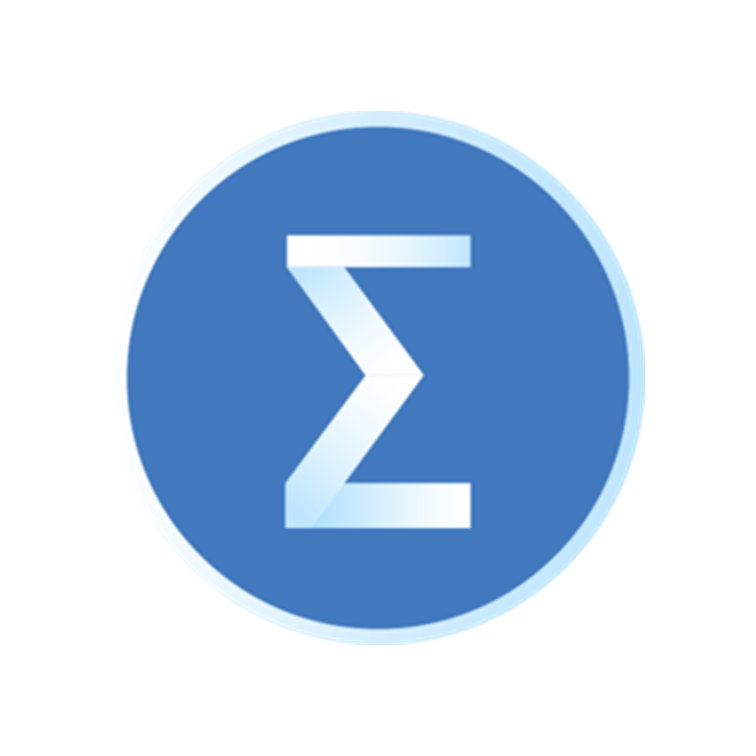
Stata 18 統計分析軟體
Stata 18 在全球被廣泛應用於企業和學術機構中。許多使用者工作在研究領域,特別是在經濟學、社會學、政治學及流行病學領域。
IRTPRO 5.1項目反應測驗軟體
IRTPRO™ 是使用項目反應理論 (IRT) 進行項目校準和測試評分的高級應用程式。它帶有直觀的圖形用戶界面,並提供內置的生產質量 IRT 圖形。IRTPRO™適用於教育工作者、學生、研究人員和評估組織,在教育、心理、社會和健康科學領域的應用越來越廣泛。
MLwiN 3.05 多層次模型軟體
MLwiN可以擬合從一般的多水平線形模型到極複雜的多水平生存分析模型、多水平時間序列模型等,幾乎已囊括多水平統計模型的全部領域,並且水平數不限!