nQuery Advisor 8.7 統計分析軟體
- nQuery Advisor 8.7 統計分析軟體
-
類別統計分析軟體
-
介紹nQuery Advisor提供一簡單可靠及有效的方法,以決定樣本的大小及統計的幕數。提供專家計算找出可靠區間及等效分析,來決定樣本的大小
nQuery Advisor 8.7 Statistical Analysis Software
nQuery release (v8.7) continues to help biostatisticians and clinical researchers
make clinical trials faster, less costly and more successful.
28 new sample size tables have been added in total
What's new in the PRO tier of nQuery?
In the Spring 2021 nQuery Advanced 8.7 release, 5 new sample size tables have been added to the Pro tier of nQuery covering various new adaptive designs.
In this release the following areas are targeted for
development:
• Multi-Arm Multi-Stage Designs (MAMs) GSD
• Two Stage Phase II Designs
• Generalized MCP-Mod (Multiple Comparisons
Procedure - Modelling)
• Group Sequential Designs
Multi-Arm Multi-Stage Designs (MAMs) GSD
Adaptive designs, such as the group sequential design, have become widely used in clinical drug development. However, much of this development has been confined to the two-arm setting. A common situation in clinical trials is that in which an optimal treatment or dose has not been identified prior to the phase III trial. In this situation, it may be desirable to begin a Phase II trial (or longer Phase III trial) with several treatment arms and conduct interim analyses that will allow the dropping of less promising arms and potentially seamlessly move those arms into Phase III. This type of design is commonly named a multi-arm multi-stage design.
The Multi Arm Multi Stage (MAMs) group sequential design extends the common two-arm group sequential design to a design in which multiple treatment arms can be compared to a common control arm and allows the trial to be stopped for efficacy or futility based on the best performing arm. In addition, it can be shown that less effective arms may be dropped at interim analyses without affecting the Type I error.
MAMs designs provide the ability to assess more treatments in less time than could be done with a series of two-arm trials and can offer significantly smaller sample size requirements when compared to that required for the equivalent number of two-arm trials.
In this release, we have extended our MAMs Group Sequential designs to include an option for the proportions endpoint. This provides a familiar framework with which to conduct a MAMs design and explore the additional flexibility offered.
The table added is as follows:
• Multiple Arms Multiple Stage (MAMs) Group Sequential
Design for Proportions
Two Stage Phase II Designs
Phase II designs are often used to determine whether a new procedure or treatment is likely to meet a basic level of efficacy to warrant further development or evaluation. Phase IIa designs are focussed on the proof-of-concept part of Phase II trials. They aim to show the potential efficacy and safety of a proposed treatment. Two-stage designs are common to allow for flexibility to stop trials early for futility as Phase II is the most common failure point in drug evaluation. We have implemented two new two-stage Phase II designs in this release.
The first follows the method outlined in Bryant and Day (1995). As mentioned, often evaluation of toxicity is listed as a primary objective in Phase IIa trials. The Bryant and Day design is a two stage phase IIa design with a coprimary endpoint which allows one to evaluate both the efficacy and the toxicity of the drug or treatment within a single design.
In addition to this design, we have also implemented an adaptive response design based on the paper by Lin & Shih (2004). The main benefit of the method put forward by Lin and Shih over methods such as Simon's two-stage design, is the way in which the proportion of successes under which the alternative hypothesis is powered can be specified. This method allows the specification of two response proportions, the first a low or pessimistic value, and the second an optimistic or high value. This allows one to combat the uncertainty in the specification of the proportion of desirable results and so mitigates the risk of having a proportion which is too high, and so results in the study being underpowered, or a proportion which is too low, and so results in patient resources being unnecessarily wasted or the study taking an unnecessarily long time to complete.
The tables added are as follows:
• Two Stage Phase II Design for Response and Toxicity
(Bryant and Day)
• Adaptive Response Two Stage Phase II Design
(Lin & Shih's Design)
MCP-Mod (Multiple Comparisons Procedure - Modelling)
MCP-Mod (Multiple Comparisons Procedure - Modelling) is an increasingly popular statistical methodology for dose-finding Phase IIb trials. Since its development at Novartis, MCP-Mod promises to devise proof-of-concept and dose-ranging trials with greater evidence and data that can prove critical for Phase III clinical trial design.
Combining the robustness of multiple comparisons procedures with the flexibility of modelling, MCP-mod combines these methods to provide superior statistical evidence from Phase II trials with regards to dose selection with the FDA & EMA approving MCP-Mod as fit-for-purpose (FFP).
In this release, we have extended our MCP-Mod design to include the case where there are unequal standard deviations for each arm in the study.
The table added is as follows:
• Multiple Comparisons Procedure - Modelling for
Continuous Outcome (Unequal Variance) (MCP-Mod)
Group Sequential Tests for Non-inferiority Two Means
Group sequential designs are a widely used type of adaptive trial in confirmatory Phase III clinical trials. Group sequential designs differ from a standard fixed term trial by allowing a trial to end early at pre-specified interim analyses for efficacy or futility. Group sequential designs achieve this by using a flexible error spending method which allows a set amount of the total Type I or Type II error at each interim analysis. The group sequential design allows the trialist the flexibility to end those trials early which otherwise would have led to another large cohort of subjects to be analysed unnecessarily.
Non-inferiority testing is used to test if a new treatment is non-inferior to a standard treatment by a pre-specified amount. This is a common objective in areas such as medical devices and generic drug development. This release sees one new design being implemented in this area.
The table added is as follows:
• Group Sequential Test for Non-inferiority of Mean Difference
List of new Adaptive tables
Phase II Multistage Designs
• Two Stage Phase II Design for Response and Toxicity
(Bryant and Day)
Proportion > Two - Test
• Multiple Arms Multiple Stage (MaMs) Group
Sequential Design for Proportions
Group Sequential Designs
• Group Sequential Test for Non-inferiority of Mean Difference
Means > Two - Test
• Multiple Comparisons Procedure - Modelling for
Continuous Outcome (Unequal Variance)
Phase II Multistage Designs
• Oncology Two Stage Phase IIA Design
What's new in the PLUS tier of nQuery?
In the Spring 2021 nQuery Advanced 8.7 release, 2 new sample size tables have been added to the Plus Tier of nQuery.
Two main areas are targeted for development. These are:
• Bayesian factors
• Consensus Based Intervals
Bayesian Factor for One Mean
Bayes factors can be described as the Bayesian equivalent of the p-value. The sample size is chosen to make it a priori probable that the Bayes factor is greater than a given cut-off of prespecificed size. Using Bayes factors allows evaluation of evidence in favor of a null hypothesis, as opposed to in frequentist settings where evidence can only be found in favor of the alternative hypothesis.
The table added is as follows:
• Bayesian Factor for One Mean
Consensus Based Intervals
Consensus based intervals two highest posterior density (HPD) credible intervals for a binomial proportion to agree to within a prespecified distance. The two intervals arise from the use of two different prior densities, each following a beta distribution. This method is useful in situations where there are multiple prior opinions about the true proportion and we wish to incorporate an “enthusiastic” and “pessimistic” prior into our calculations.
The table added is as follows:
• Bayesian Consensus-Based Sample Size for One Proportion
List of new Bayesian tables
Means - One - Test
• Bayesian Factor for One Mean
Proportions One Confidence Interval
• Bayesian Consensus-Based Sample Size for One Proportion
What's new in the BASE tier of nQuery?
In the Spring 2021 nQuery Advanced 8.7 release, 21 new sample size tables have been added to nQuery. This release summary will provide an overview of which areas have been targeted along with the full list of tables added. The main areas targeted for development are as follows:
• Tests for Proportions
• Survival/Time to Event Trials
Tests for Proportions
Proportions are a common type of data where the most common endpoint of interest is a dichotomous variable. Examples in clinical trials include the proportion of patients who experience a tumour regression. There are a wide variety of designs proposed for binary proportions ranging from exact to maximum likelihood to normal approximations.
Under this broad area of proportions we have added tables across the areas of inequality, equivalence and non-inferiority testing. The tables added under this general heading are as follows:
• Inequality Tests for One Proportion
• Non-Inferiority Test for Difference in Paired Proportions
• Non-Inferiority Test for Ratio of Paired Proportions
• Inequality Tests for Difference of Two Proportions
• Inequality Tests for Ratio of Two Proportions
• Inequality Tests for Odds Ratio of Two Proportions
• Equivalence Tests for Ratio of Two Proportions
• Equivalence Tests for Odds Ratio of Two Proportions
In addition to these more general tables we have also added
some more specialised Proportions tables
The additional tables added fall under the following categories:
• Cross-over designs
• Reliability Demonstration Tests
• Shedding Studies
Cross-over Designs
In a crossover design each subject receives all treatments at least once with the objective of measuring study differences among the treatments. “Crossover” comes from the most common two treatments case which is of interest here. Crossover designs are popular due to an expected increase in precision, since each subject effectively acts as their own control, and the concomitant reduction in study size but do have additional statistical and practical complications such as carry-over effects.
The tables added are as follows:
• Inequality Test for the Difference of Two Proportions in
a 2x2 Crossover Design
• Inequality Test for the Odds Ratio of Two Proportions in
a 2x2 Crossover Design
• Equivalence Test for the Difference of Two Proportions in
a 2x2 Crossover Design
• Equivalence Test for the Odds Ratio of Two Proportions in
a 2x2 Crossover Design
• Non-inferiority Test for the Difference of Two Proportions in
a 2x2 Crossover Design
• Non-inferiority Test for the Odds Ratio of Two Proportions in
a 2x2 Crossover Design
Reliability Demonstration Tests
A reliability demonstration test is one which deals with the area of rare binomial events and tests the minimum required proportion of successes, known as the reliability, against a standard level. These tests are useful where a manufacturer will have to demonstrate that a certain product has met a goal of a certain reliability at a given time with a specific level of confidence.
• Test to Demonstrate Reliability for One Proportion
• Test to Demonstrate Reliability for One Proportion
with Specified Adverse Events
Shedding Studies
Shedding studies relate to studies with a binomial outcome consisting of repeated binary measures with autocorrelation over time. This type of outcome is common in viral shedding studies, in which each individual's outcome is a proportion. However, these methods can be applied to any binomial outcome in a longitudinal assessment of an intermittent event.
The tables added are as follows:
• Test for Autocorrelated Proportion Cohort Design
(Shedding Study)
• Test for Autocorrelated Proportion Cohort Design
(Shedding Study)
Survival/Time to Event Trials
Survival or Time-to-Event trials are trials in which the endpoint of interest is the time until a particular event occurs, for example death or tumour regression. Survival analysis is often encountered in areas such as oncology or cardiology. In contrast to studies with non-survival based endpoints, like continuous or binary endpoints for example, the statistical power of a time-to-event study is determined, not by the number of subjects in the study, but rather by the number of events which are observed and this requires additional flexibility when designing and analyzing such trials.
The survival tables being added this release fall into the following categories:
• Log-Rank test
• Piecewise Weighted Log-Rank Test for Delayed Effect
• Restricted Mean Survival Times
Log-Rank Test
The log-rank test is one of the most common statistical tests used for the analysis of survival data. Its flexibility and interpretability provide useful insights into the comparable hazard rates in survival trials.
In this release one new table is being added for the log rank test. It allows for a constant accrual rate for the two sample log-rank test. Where it is assumed that both the event rates and the dropouts follow an exponential distribution. This additional option provides more flexibility in the area of the log-rank test.
The table added is as follows:
• Two Sample Log-Rank Test Assuming Constant Accrual,
Exponential Rates, Dropouts
Piecewise Weighted Log-Rank Test for Delayed Effect
There is increasing interest in survival analysis for flexible models due to the increasing occurrence of non-proportional hazards in clinical trials in areas such as immunotherapy. The piecewise weighted log-rank test has been proposed as one potential method that allows a survival analysis to account for a delayed effect. This method splits the trial into two or more time periods which are weighted to create a better estimate of the overall effect by accounting for the different effect sizes expected during different time intervals.
In this release, the Analytic Power Calculation Method based on Generalised Piecewise Weighted Log-rank Test (APPLE+) method approach for determining the sample size for a weighted log-rank test for a delayed effect is added. This method is useful in detecting a difference between two hazard functions when there is a time-lag to treatment effect. This results in a delayed separation of the survival curves for the experimental and control groups, so the proportional hazard assumption no longer holds. This type of design is often encountered in immunotherapy trials when the immune system takes time to respond to the treatment.
In order to account for variations in the delayed onset of clinical effect between patients, the time for treatment to take effect occurs randomly between user-specified upper and lower time bounds. We assume that there is no treatment effect before this time and that the treatment is fully effective after this time. The calculation uses the APPLE+ (Analytic Power Calculation Method based on Generalised Piecewise Weighted Log-rank Test) method as described by Xu et al. (2018).
The table added is as follows:
• Generalized Piecewise Weighted Log-rank Test with
random treatment time lag effect Survival Model
(APPLE+ Method)
List of new BASE Tables
Proportions One Test
• Test to Demonstrate Reliability for One Proportion
• Test to Demonstrate Reliability for One Proportion
with Specified Adverse Events
• Inequalty Tests for One Proportion
Prop - One - Non-inferiority
• Non-Inferiority Test for Difference in Paired Proportions
• Non-Inferiority Test for Ratio of Paired Proportions
Prop - Two - Test
• Inequality Test for the Difference of Two Proportions in
a 2x2 Crossover Design
• Inequality Test for the Odds Ratio of Two Proportions in
a 2x2 Crossover Design
• Test for Autocorrelated Proportion Cohort Design
(Shedding Study)
• Test for Autocorrelated Proportion Crossover Design
(Shedding Study)
• Inequality Tests for Difference of Two Proportions
• Inequality Tests for Ratio of Two Proportions
• Inequality Tests for Odds Ratio of Two Proportions
Prop - Two - Equivalence
• Equivalence Test for the Difference of Two Proportions in
a 2x2 Crossover Design
• Equivalence Test for the Odds Ratio of Two Proportions in
a 2x2 Crossover Design
• Equivalence Tests for the Ratio of Two Proportions
• Equivalence Tests for the Odds Ratio of Two Proportions
Prop - Two - NI
• Non-inferiority Test for the Difference of Two Proportions in a 2x2 Crossover Design
• Non-inferiority Test for the Odds Ratio of Two Proportions in
a 2x2 Crossover Design
Survival - Two - Test
• Two Sample Log-Rank Test Assuming Constant Accrual,
Exponential Rates, Dropouts
• Generalised Piecewise Weighted Log-rank Test with
Random Treatment Time Lag Effect Survival Model
(APPLE+ Method)
Survival - Two - NI
• Non-Inferiority Test for the Difference of Two Restricted
Mean Survival Times
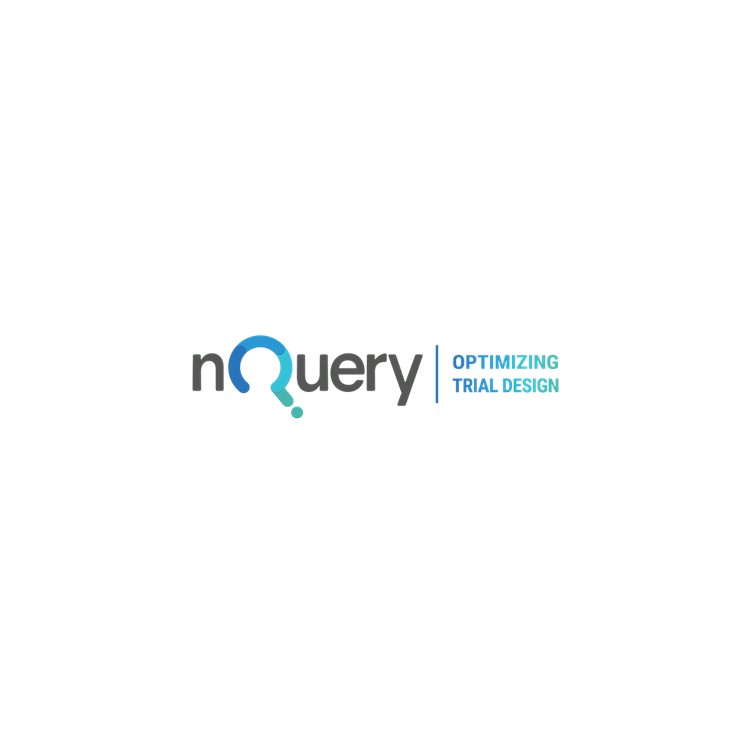
系統需求
Windows 10, then it must be higher than version 1511.
-Please note that Windows 10 S is not supported.
CPU:
2 core processor of 1 GHz 'speed'
HD:
3 GB of available hard disk space
RAM:
2GB RAM
Other:
.NET 框架 4.7.1
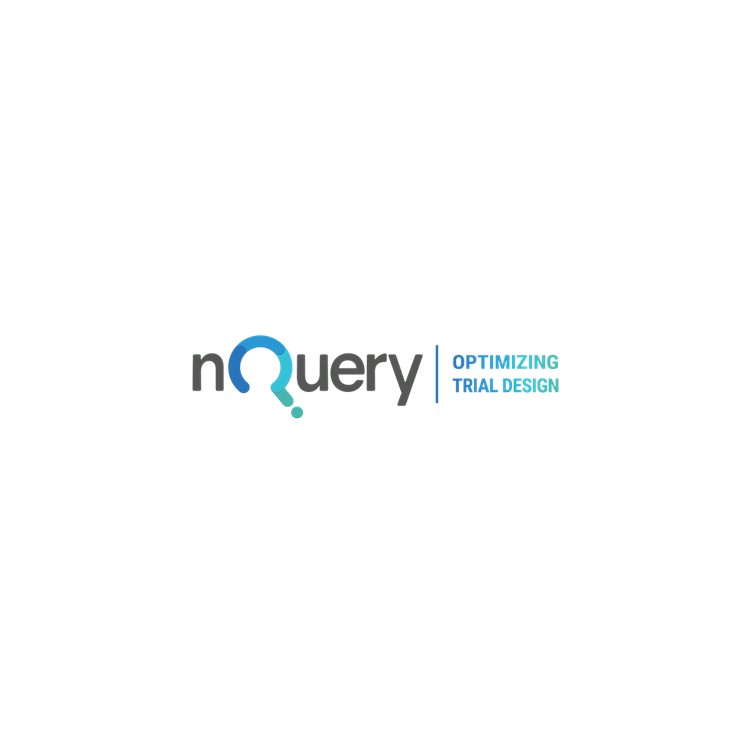
nQuery Advisor 8.7 統計分析軟體
nQuery 版本 (v8.7) 繼續幫助生物統計學家和臨床研究人員
使臨床試驗更快、成本更低、更成功。 總共增加了28 個新的樣本量表
nQuery 的 PRO 層有什麼新功能?
在 Spring 2021 nQuery Advanced 8.7 版本中,nQuery的 Pro 層添加了 5 個新的樣本大小表,涵蓋了各種新的自適應設計。
在此版本中,以下區域是針對開發的:
• 多臂多階段設計 (MAM) GSD
• 兩階段二期設計
• 廣義 MCP-Mod(多重比較程序 - 建模)
• 分組順序設計
多臂多階段設計 (MAM) GSD
適應性設計,如組序貫設計,已廣泛應用於臨床藥物開發。然而,大部分這種發展僅限於兩臂設置。臨床試驗中的常見情況是在 III 期試驗之前尚未確定最佳治療或劑量。在這種情況下,可能需要對多個治療組開始 II 期試驗(或更長的 III 期試驗),並進行中期分析,以允許放棄不太有希望的治療組,並有可能將這些治療組無縫地轉移到 III 期。這種類型的設計通常稱為多臂多級設計。
多臂多階段 (MAM) 組序貫設計將常見的兩臂組序貫設計擴展到這樣一種設計,其中多個治療組可以與一個普通對照組進行比較,並允許基於有效性或無效性停止試驗表現最好的手臂。此外,可以證明,在不影響 I 類錯誤的情況下,在中期分析中可能會放棄效率較低的武器。
與一系列雙臂試驗相比,MAM 設計能夠在更短的時間內評估更多治療,並且與同等數量的雙臂試驗所需的樣本量相比,可以提供顯著更小的樣本量要求。
在此版本中,我們擴展了 MAMs Group Sequential 設計,以包含一個比例端點選項。這提供了一個熟悉的框架,用於進行 MAM 設計並探索所提供的額外靈活性。
添加的表格如下:
• 多臂多級 (MAM) 組按比例順序設計
兩階段二期設計
II 期設計通常用於確定新程序或治療是否可能滿足基本療效水平以保證進一步開發或評估。IIa 期設計側重於 II 期試驗的概念驗證部分。他們旨在展示擬議治療的潛在功效和安全性。兩階段設計很常見,以允許靈活地提前停止試驗,因為 II 期是藥物評估中最常見的失敗點。我們在此版本中實施了兩個新的兩階段階段 II 設計。
第一個遵循 Bryant 和 Day (1995) 中概述的方法。如上所述,通常將毒性評估列為 IIa 期試驗的主要目標。Bryant 和 Day 設計是一個具有共同主要終點的兩階段 IIa 期設計,允許在單一設計中評估藥物或治療的功效和毒性。
除了這種設計,我們還基於 Lin & Shih (2004) 的論文實施了自適應響應設計。Lin 和 Shih 提出的方法相對於 Simon 的兩階段設計等方法的主要好處是可以指定替代假設有效的成功比例的方式。此方法允許指定兩個響應比例,第一個是低值或悲觀值,第二個是樂觀值或高值。這使人們能夠應對理想結果比例規範中的不確定性,從而降低比例過高的風險,從而導致研究動力不足,或比例過低,
添加的表如下:
• 響應和毒性的兩階段 II 期設計(Bryant 和 Day)
• 自適應響應二階段二期設計(Lin & Shih's Design)
MCP-Mod(多重比較程序 - 建模)
MCP-Mod(多重比較程序 - 建模)是一種越來越流行的用於劑量發現 IIb 期試驗的統計方法。自在諾華開發以來,MCP-Mod 承諾設計概念驗證和劑量範圍試驗,並提供更多證據和數據,這些證據和數據對 III 期臨床試驗設計至關重要。
將多重比較程序的穩健性與建模的靈活性相結合,MCP-mod 將這些方法結合起來,從 II 期試驗中提供關於劑量選擇的卓越統計證據,FDA 和 EMA 批准 MCP-Mod 為適合用途 (FFP) )。
在此版本中,我們擴展了我們的 MCP-Mod 設計,以包括研究中每個臂的標準偏差不相等的情況。
添加的表格如下:
• 多重比較程序 - 連續結果建模(不等方差)(MCP-Mod)
非劣效性二均值的組序檢驗
組序貫設計是驗證性 III 期臨床試驗中廣泛使用的適應性試驗類型。組序貫設計與標準的固定期限試驗不同,它允許試驗在預先指定的中期療效或無效分析中提前結束。組序貫設計通過使用靈活的錯誤消耗方法來實現這一點,該方法允許在每次中期分析時設定一定數量的 I 類或 II 類總錯誤。組序貫設計允許試驗者靈活地提前結束這些試驗,否則會導致對另一大組受試者進行不必要的分析。
非劣效性測試用於測試新治療是否在預先指定的量上不劣於標準治療。這是醫療器械和仿製藥開發等領域的共同目標。此版本將在該領域實施一項新設計。
添加的表格如下:
• 平均差非劣效性的組序檢驗
新的自適應表列表
第二階段多級設計
• 響應和毒性的兩階段 II 期設計(Bryant 和 Day)
比例 > 2 - 測試
• 多臂多級 (MaMs) 組按比例順序設計
分組順序設計
• 平均差非劣效性的組序檢驗
均值 > 2 - 檢驗
多重比較程序 - 連續結果建模(不等方差)
第二階段多級設計
• 腫瘤學兩階段 IIA 設計
nQuery PLUS 層有哪些新功能?
在 Spring 2021 nQuery Advanced 8.7 版本中,nQuery 的 Plus Tier 添加了 2 個新的樣本大小表。
兩個主要領域是發展的目標。這些都是:
• 貝葉斯因子
• 基於共識的區間
一個均值的貝葉斯因子
貝葉斯因子可以描述為p值的貝葉斯等價物。選擇樣本大小是為了使貝葉斯因子大於預先指定大小的給定截止值的先驗概率。
使用貝葉斯因子允許評估有利於零假設的證據,而不是在頻率論環境中只能找到支持替代假設的證據。
添加的表格如下:
• 一個均值的貝葉斯因子
基於共識的區間
基於共識的區間兩個最高後驗密度 (HPD) 可信區間,用於在預先指定的距離內同意二項式比例。這兩個區間源於使用兩個不同的先驗密度,每個密度都遵循 beta 分佈。這種方法在關於真實比例有多種先驗意見的情況下很有用,我們希望將“熱情”和“悲觀”先驗納入我們的計算中。
添加的表格如下:
• 一個比例的基於貝葉斯共識的樣本量
新貝葉斯表列表
手段 - 一 - 測試
• 一個均值的貝葉斯因子
一個置信區間的比例
• 一個比例的基於貝葉斯共識的樣本量
nQuery BASE 層有什麼新變化?
在 Spring 2021 nQuery Advanced 8.7 版本中,nQuery 中添加了21 個新的樣本大小表。此版本摘要將概述已針對哪些區域以及添加的表格的完整列表。主要開發領域如下:
• 比例測試
• 生存/事件試驗時間
比例測試
比例是一種常見的數據類型,其中最常見的感興趣端點是二分變量。臨床試驗中的例子包括經歷腫瘤消退的患者比例。對於二進制比例提出了各種各樣的設計,範圍從精確到最大似然再到正態近似。
在這個廣泛的比例領域下,我們在不平等、等價性和非劣效性測試領域添加了表格。在這個總標題下添加的表格如下:
• 一個比例的不等式檢驗
• 配對比例差異的非劣效性檢驗
• 配對比例的非劣效性檢驗
• 兩個比例差的不等式檢驗
• 兩個比例之比的不等式檢驗
• 兩個比例的優勢比的不等式檢驗
• 兩個比例之比的等效檢驗
• 兩個比例的優勢比的等效檢驗
除了這些更通用的表格之外,我們還添加了一些更專業的比例表格
添加的附加表屬於以下類別:
• 交叉設計
• 可靠性演示測試
• 脫落研究
交叉式設計小號
在交叉設計中,每個受試者至少接受一次所有治療,目的是測量治療之間的研究差異。“交叉”來自最常見的兩種治療案例,這裡很有趣。由於預期的精確度增加,交叉設計很受歡迎,因為每個受試者有效地充當他們自己的對照,並且研究規模隨之減少,但確實有額外的統計和實際並發症,例如遺留效應。
添加的表如下:
• 2x2 交叉設計中兩個比例差異的不等式檢驗
• 2x2 交叉設計中兩個比例的優勢比的不等式檢驗
• 2x2 交叉設計中兩個比例差異的等效性檢驗
• 2x2 交叉設計中兩個比例的優勢比的等效檢驗
• 2x2 交叉設計中兩個比例差異的非劣效性檢驗
• 2x2 交叉設計中兩個比例的優勢比的非劣效性檢驗
可靠性演示測試
可靠性演示測試是一種處理罕見二項式事件區域並針對標準水平測試所需的最小成功比例(稱為可靠性)的測試。這些測試在製造商必須證明某個產品在給定時間以特定的置信度達到一定可靠性的目標時非常有用。
• 測試以證明一部分的可靠性
• 測試以證明具有特定不良事件的一部分的可靠性
脫落研究
脫落研究涉及二項式結果的研究,該結果由隨時間具有自相關性的重複二元測量組成。這種類型的結果在病毒脫落研究中很常見,其中每個人的結果都是一個比例。然而,這些方法可以應用於間歇性事件的縱向評估中的任何二項式結果。
添加的表如下:
• 自相關比例隊列設計檢驗(脫落研究)
• 自相關比例交叉設計的檢驗(脫落研究)
生存/事件試驗時間
生存或事件時間試驗是其中感興趣的終點是特定事件發生之前的時間的試驗,例如死亡或腫瘤消退。生存分析經常在腫瘤學或心髒病學等領域遇到。與基於非生存期終點的研究(例如連續或二元終點)相比,事件發生時間研究的統計功效不是由研究中的受試者數量決定的,而是由事件數量決定的這在設計和分析此類試驗時需要額外的靈活性。
此版本中添加的生存表分為以下幾類:
• 對數秩檢驗
• 延遲效應的分段加權對數秩檢驗
• 受限制的平均生存時間
對數秩檢驗
對數秩檢驗是用於分析生存數據的最常見的統計檢驗之一。它的靈活性和可解釋性為了解生存試驗中的可比風險率提供了有用的見解。
在此版本中,為日誌等級測試添加了一個新表。它允許兩個樣本對數秩檢驗具有恆定的應計率。假設事件率和輟學率都遵循指數分佈。此附加選項在對數秩檢驗領域提供了更大的靈活性。
添加的表格如下:
• 兩個樣本對數秩檢驗假設恆定應計、指數率、輟學
延遲效應的分段加權對數秩檢驗
由於在免疫治療等領域的臨床試驗中越來越多地出現非比例風險,因此對靈活模型的生存分析越來越感興趣。分段加權對數秩檢驗已被提議作為一種允許生存分析來解釋延遲效應的潛在方法。這種方法將試驗分成兩個或多個時間段,通過考慮不同時間間隔內預期的不同效果大小,對這些時間段進行加權以更好地估計整體效果。
在此版本中,添加了基於廣義分段加權對數秩檢驗 (APPLE+) 方法的分析功效計算方法,用於確定延遲效應加權對數秩檢驗的樣本量。當治療效果存在時滯時,此方法可用於檢測兩個危險函數之間的差異。這導致實驗組和對照組的生存曲線延遲分離,因此比例風險假設不再成立。當免疫系統需要時間來響應治療時,在免疫治療試驗中經常會遇到這種類型的設計
為了解決患者之間臨床效應延遲發生的差異,治療生效的時間在用戶指定的時間上限和下限之間隨機發生。我們假設在此時間之前沒有治療效果,而在此時間之後治療完全有效。該計算使用了 Xu 等人所述的 APPLE+(基於廣義分段加權對數秩檢驗的分析功效計算方法)方法。(2018)。
添加的表格如下:
• 具有隨機治療時滯效應生存模型的廣義分段加權對數秩檢驗
(APPLE+ 方法)
新 BASE 表列表
比例一測試
• 測試以證明一部分的可靠性
• 測試以證明具有特定不良事件的一部分的可靠性
• 一個比例的不等式檢驗
道具 - 一 - 非劣效性
• 配對比例差異的非劣效性檢驗
• 配對比例的非劣效性檢驗
道具 - 二 - 測試
• 2x2 交叉設計中兩個比例差異的不等式檢驗
• 2x2 交叉設計中兩個比例的優勢比的不等式檢驗
• 自相關比例隊列設計檢驗(脫落研究)
• 自相關比例交叉設計的檢驗(脫落研究)
• 兩個比例差的不等式檢驗
• 兩個比例之比的不等式檢驗
• 兩個比例的優勢比的不等式檢驗
Prop - 二 - 等價
• 2x2 交叉設計中兩個比例差異的等效性檢驗
• 2x2 交叉設計中兩個比例的優勢比的等效檢驗
• 兩個比例之比的等效檢驗
• 兩個比例的優勢比的等價檢驗
道具 - 兩個 - NI
• 2x2 交叉設計中兩個比例差異的非劣效性檢驗
• 2x2 交叉設計中兩個比例的優勢比的非劣效性檢驗
生存 - 二 - 測試
• 兩個樣本對數秩檢驗假設恆定應計、指數率、輟學
• 具有隨機處理時間滯後效應生存模型的廣義分段加權對數秩檢驗
(APPLE+ 方法)
生存 - 兩個 - NI
• 兩個受限平均生存時間差異的非劣效性檢驗
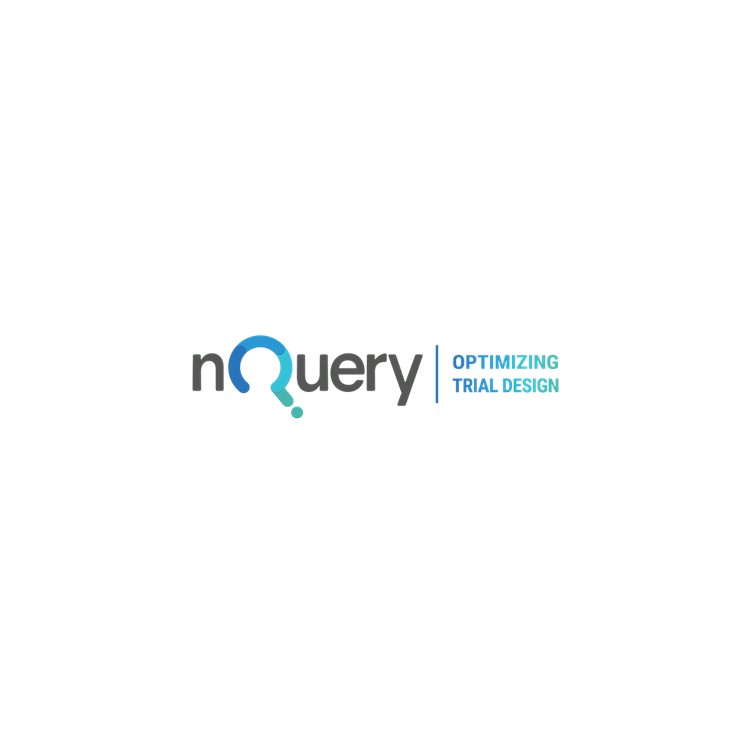
PASS 2022 統計分析軟體
PASS 軟體為超過 1100 個統計測試和置信區間場景提供樣本大小工具 -是任何其他樣本大小軟體的兩倍多。每個工具都經過了已發表的文章和/或文字的仔細驗證。 PASS 配備整合文件與PhD統計人員支援。 PASS 經過 20 多年的微調,現已成為臨床試驗、製藥和其他醫學研究的領先樣本量軟體選擇。它還已成為所有其他需要樣本量計算或評估的領域的支柱。
IBM SPSS AMOS 結構方程模型分析軟體
IBM SPSS AMOS 是一款專業的結構方程模型(SEM)分析軟體,可用於數據分析、統計建模、因果關係分析等。該軟體基於最新的結構方程建模技術,可幫助用戶構建複雜的模型並進行分析。您可以繪製結構方程模型圖、設置模型參數、模型適配度檢測、多樣本比較等操作。該軟體支持多種分析方法,如路徑分析、因果關係分析、驗證性因子分析、多層次建模等,幫助用戶從數據中發現深層次的因果關係和影響因素。此外,IBM SPSS AMOS 還提供了豐富的圖表和報告生成工具,使得用戶可以直接地呈現和分享研究成果。如果您是一名統計學家、社會科學研究人員、市場調研人員或學術人員,IBM SPSS AMOS 將是您不可或缺的工具。
XpertRule® Miner 資料採礦軟體
XpertRule Miner所採用之資料採礦(data mining)是最近學術界與產業界十分重視的技術,它是藉由資訊技術從大量資料裡粹取出資訊與結果之間的關聯性。企業可以用它得到顧客與購買產品之間的相關資訊。現今大多數企業在分析他們的客戶資料時,採取傳統統計方法所做出的結果,仍具有改進的空間。