HB-Reg v4.2.2303 貝斯階層回歸軟體
- HB-Reg v4.2.2303 貝斯階層回歸軟體
-
類別研究分析軟體
-
介紹什麼是分層貝斯階層回歸(HB-Reg)?在市場研究數據的分析中,有很多時候研究人員有受訪者,商店或其他實驗單位的樣本,並希望估計每個單位的單獨回歸係數。在過去幾年中,分層貝葉斯(HB)估計在市場營銷研究中發揮著越來越重要的作用。它可以在精度和有效性方面改進參數估計(例如β權重和效用)。我們的HB-Reg軟件用於基於回歸的問題,其中受訪者提供包含連續因變量的多個觀察(病例)。
HB-Reg v4.2.2303 Bayside regression software
HB-Reg software is a general analytical tool for estimating regression problems at the individual level involving a continuous dependent variable. It is only appropriate when respondents provide multiple observations (cases).
Features
•Up to 1000 parameters per respondent, for unlimited respon
dents.
•Uses comma-delimited input files
•HB-Reg is designed to run on Microsoft Windows 2000 or
later.
Methodology
In the analysis of marketing research data, there are many occasions when the researcher has a sample of respondents, stores, or other experimental units, and wishes to estimate separate regression coefficients for each unit.
Consider three examples:
In full-profile conjoint analysis, respondents give preference ratings for hypothetical product concepts. Regression analysis is often used, where the independent variables are columns of a "design matrix" describing the concepts, and the dependent variable consists of preference ratings.
Respondents in a customer satisfaction study provide ratings of several companies. Some ratings are on "explanatory" variables, such as customer service, product durability, convenience of use, etc. Other ratings are more general, such as overall satisfaction with the companies' products. One goal of the study is to infer the relative importance of each explanatory factor in determining overall satisfaction.
During a pricing experiment in grocery stores, the prices of several products are varied systematically in different time periods, and sales of each product are measured with scanner data. The independent variables are product prices and other factors such as the presence of displays, coupons, and newspaper features. The dependent variables are product sales.
In each situation, respondents or stores may have different regression functions. In the past, researchers have often tried to handle this problem by ignoring heterogeneity among individuals, pooling all the data, and estimating a single set of regression coefficients that describe the "average" individual. However, aggregate regression confounds heterogeneity (true differences between respondents/stores) with noise. Because HB-Reg can distinguish heterogeneity from noise, it results in more stable individual- AND aggregate-level estimates of betas. HB-Reg also is more robust in the case of multicolinearity among the independent variables than aggregate regression.
Several recent articles have shown that hierarchical Bayes estimation can do a creditable job of estimating individual parameters even when there are more parameters than observations per individual. This is done by considering each individual to be a sample from a population of similar individuals, and "borrowing" information from other individuals in the estimation for each one.
HB-Reg offers parameter constraints, meaning the ability to constrain certain parameters to be larger (smaller) than others, or to be greater than or less than zero. Advanced users can also control the prior variance and covariances, and degrees of freedom for the prior covariance matrix. These features will permit more reasonable estimation of parameters, even when relatively sparse information is available within the unit of analysis.
When using the full-size system, up to 1000 parameters per individual can be estimated. HB-Reg requires the Microsoft .NET framework.
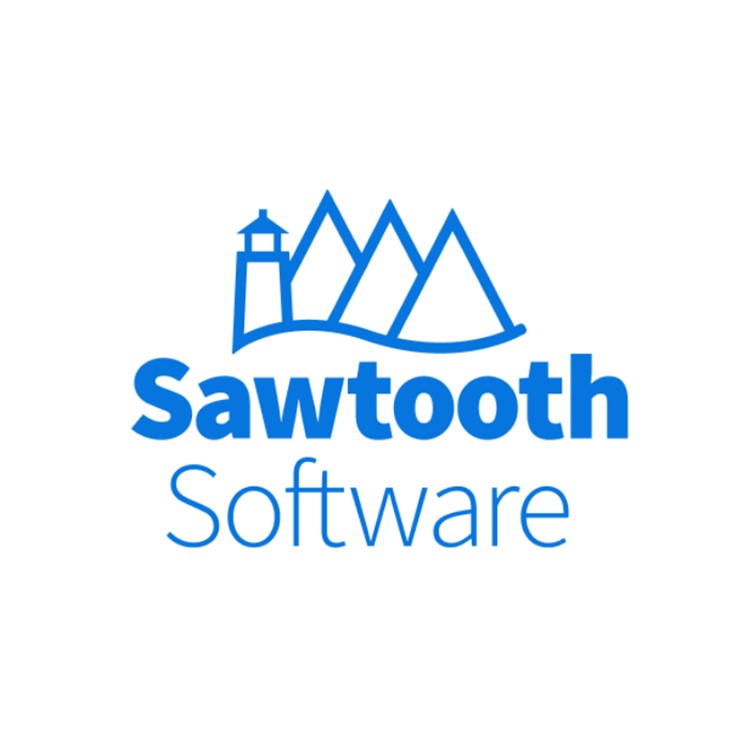
系統需求
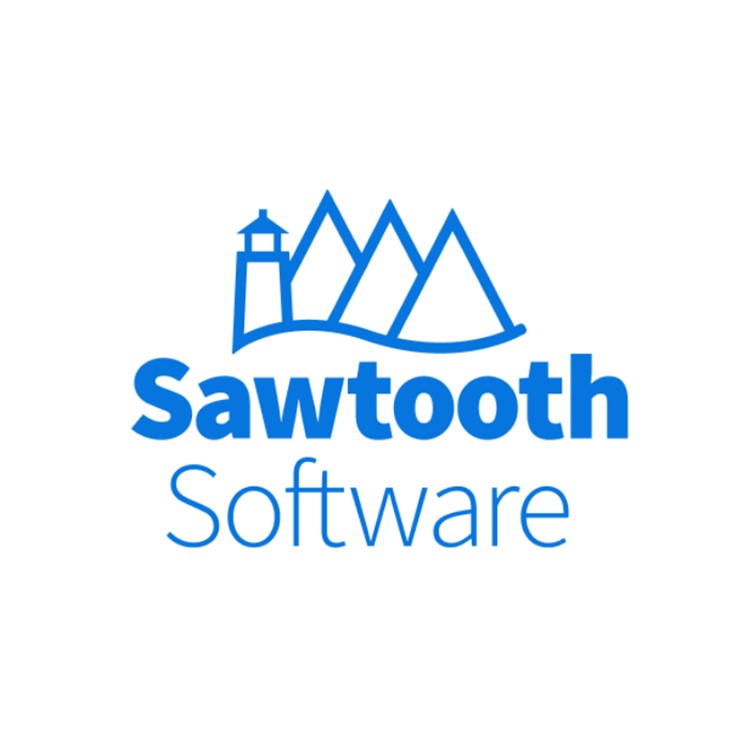
HB-Reg v4.2.2303 貝斯階層回歸軟體
HB-Reg軟件是用於評估涉及連續因變量的個體水平上回歸問題的通用分析工具。僅當受訪者提供多個觀察結果(案例)時才適用。
特徵
•每個受訪者最多可使用1000個參數,適用於無限個受訪者。
•使用逗號分隔的輸入文件
•HB-Reg設計為在Microsoft Windows 2000或更高版本上運行。
方法
在市場研究數據的分析中,很多情況下,研究人員都有受訪者,商店或其他實驗單位的樣本,並希望為每個單位估計單獨的回歸係數。
考慮以下三個示例:
在全面剖析的聯合分析中,受訪者對假設的產品概念給出了偏好等級。經常使用回歸分析,其中自變量是描述概念的“設計矩陣”的列,而因變量由偏好等級組成。
客戶滿意度研究中的受訪者提供了幾家公司的評級。一些等級是關於“解釋性”變量的,例如客戶服務,產品耐用性,使用方便性等。其他等級則更一般,例如對公司產品的總體滿意度。該研究的一個目標是推斷每個解釋因素在確定總體滿意度中的相對重要性。
在雜貨店的定價實驗中,幾種產品的價格在不同的時間段內系統地變化,並且每種產品的銷售額都使用掃描儀數據來衡量。自變量是產品價格和其他因素,例如顯示器,優惠券和報紙特徵的存在。因變量是產品銷售。
在每種情況下,受訪者或商店可能具有不同的回歸函數。過去,研究人員經常嘗試通過忽略個體之間的異質性,合併所有數據並估計描述“平均”個體的一組回歸係數來處理此問題。但是,聚集回歸將異質性(受訪者/商店之間的真實差異)與噪聲混淆。因為HB-Reg可以區分異質性與噪聲,所以它可以使beta的個體和集合級估計更加穩定。在自變量之間存在多重共線性的情況下,HB-Reg也比聚合回歸更健壯。
最近的幾篇文章表明,即使每個個體的參數多於觀測值,分層貝葉斯估計也可以在估計各個參數方面做得不錯。通過將每個個體視為一組相似個體的樣本,並從其他個體“借用”信息來估算每個個體來完成此操作。
HB-Reg提供參數約束,這意味著可以將某些參數限制為大於(小於)其他參數,或者大於或小於零。高級用戶還可以控制先驗方差和協方差,以及先驗方差矩陣的自由度。這些功能將允許對參數進行更合理的估計,即使在分析單位內可獲得相對稀疏的信息時也是如此。
使用全尺寸系統時,每個人最多可以估計1000個參數。HB-Reg需要Microsoft .NET框架。
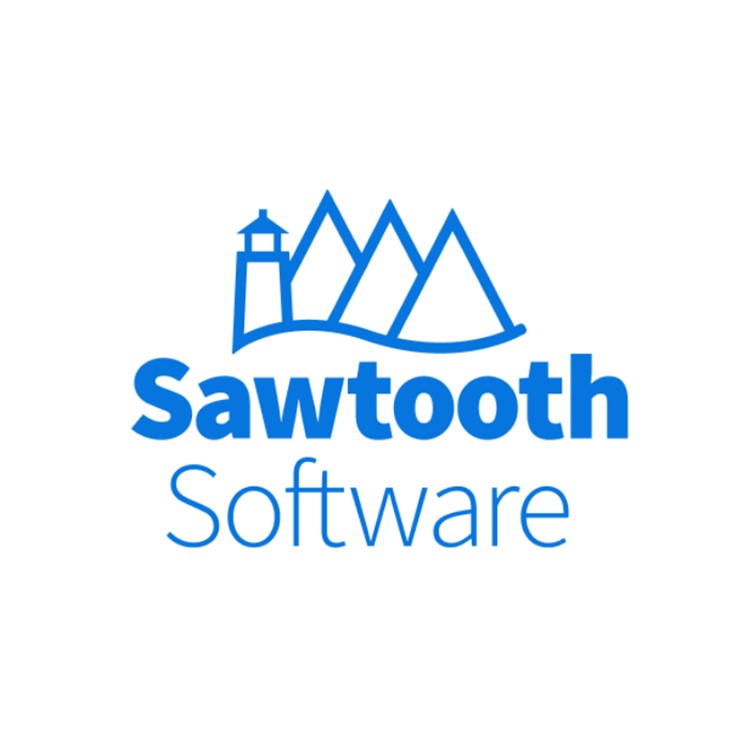
Comparion 決策支援分析軟體
Comparion Core是一個面向決策者的協作式網絡應用。它簡單易用,直觀的工作流程允許團隊定義決策標準並確定潛在的解決方案。 Comparion Core 可以追踪所有參與者的判斷、數據和評論--讓團隊專注於目標、分析和結果。
CAFE 實驗計劃法軟體
實驗計劃法軟體CAFE (Computer-Aided Formula Environment)為一套實驗設計軟體,可靠的模型驗證方式 :傳統的DOE或田口方法不區分樣本內、樣本外實驗數據之作法,其誤差被嚴重低估。CAFE採用交叉驗證法,可以在不需要增加實驗數目下,達到區分樣本內、樣本外的效果,使誤差能被準確估計。
WAsP 12 風電場設計優化與風資源預測評估軟體
丹麥Riso國家實驗室研製的WAsP軟體是目前國際認可的進行風電廠發電量計算與風機最優化佈置通用軟體。WAsP軟件套件是風力渦輪機和風電場的風資源評估,選址和能量產出計算的行業標準。WAsP軟件套件用於遍布全球各種地形的站點。對於水平和垂直外推,WAsP使用內置線性IBZ模型,該模型將適用於平坦到中等複雜的地形。如果地形非常複雜且有許多陡坡,WAsP也可以輕鬆訪問外部最先進的CFD模型。